When should you use multivariate testing, and when is A/B/n testing best?
The answer is both simple and complex.
Of course, A/B testing is the default for most people, as it is more common in optimization. But there is a time and a place for multivariate testing (MVT) as well, and it can add a lot of value.
Before we get into the nuances, let’s briefly go over the differences.
Table of contents
What is multivariate testing
Multivariate testing is a method that tests more than one element at a time on a website, in a live environmnet.
As Lars Nielsen of Sitecore explains:
Multivariate testing opposes the traditional scientific notion. Essentially, it can be described as running multiple A/B/n tests on the same page, at the same time
Multivariate testing is, in a sense, a more complex form of testing than A/B testing. A/B testing is fairly straightforward:

You can also measure the performance of three or more variations of a page with A/B/n tests. As Yaniv Navot of Dynamic Yield wrote, “High-traffic sites can use this testing method to evaluate performance of a much broader set of variations and to maximize test time with faster results.”
Here’s what an A/B/C/D test looks like conceptually:
A/B testing usually involves less combinations with more extreme changes, whereas multivariate tests have a large number of variations that usually have subtle differences.
The case for A/B/n tests
Should you use MVT or A/B/n tests?
If you have enough traffic, use both. They both serve different, but important purposes. In general, A/B tests should be your default, though.
With A/B testing, you can:
- You can test more dramatic design changes;
- Tests usually take way less time than MVTs;
- Advanced analytics can be installed and evaluated for each variation (e.g., mouse tracking info, phone call tracking, analytics integration, etc.);
- Individual elements and interaction effects can still be isolated for learning and customer theory building;
- A/B tests typically bring bigger gains (since you often test bigger changes).
A/B testing tends to get meaningful results faster. The changes between pages are more drastic, so it’s easier to tell which page is more effective.
So A/B testing harnesses the power of large changes, not just tweaking colors or headlines as is sometimes the case with MVT. Optimizers usually start all engagements with A/B testing, because that’s where the bigger gains are possible.
Yaniv Navot, Director of Online Marketing at Dynamic Yield, also mentioned that MVT is mainly used for smaller tweaks. He also mentioned that A/B tests are better for multi-page and multi-scenario experiences:
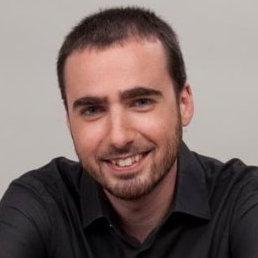
Yaniv Navot:
“Multivariate testing tends to encourages marketers to focus on small elements with little or no impact at all. Instead, marketers should focus on running programmatic and dynamic A/B tests that enable them to serve segmented experiences to multiple cohorts across the site. This cannot be achieved using traditional multivariate testing.”
Something else to worry about with MVT: the amount of traffic you get.
How much traffic do you get?
Because of the additional variations, multivariate tests require a lot of traffic. If not high traffic, at least high conversion rates.
For example, a 3×2 test (testing two different versions of three design elements) would require the same amount of traffic as an A/B test with nine variations (3^2). 3×2 is a typical MVT test.
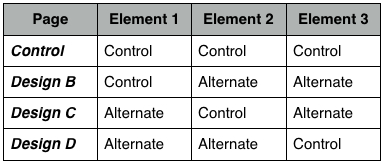
In a full factorial multivariate test, your traffic is divided evenly among all variations, which multiplies the amount of traffic necessary for statistical significance. As Leonid Pekelis, statistician at Optimizely, said, this results in a longer test run:
Altogether, the main requirement becomes running your multivariate test long enough to get enough visitors to detect many, possibly nuanced interactions.
Claire Vo of Optimizely also said that MVT is more difficult to execute because of the extra traffic and resources it requires:
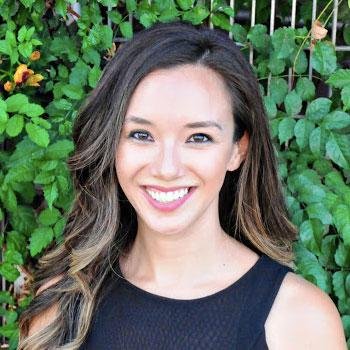
Claire Vo:
“MVT tests require significantly more investment on the technology, design, setup, and analysis side, and certainly full-factorial MVT testing can burn through significant traffic (if you even have the traffic to support this testing method). This means MVT testing can be a big burden on your conversion “budget”–whether that’s time, people, resources, or internal support.”
A rule of thumb: if your traffic is under 100,000 uniques/month, you’re probably better off doing A/B testing instead of MVT. The only exception would be the case where you have high-converting (10% to 30% conversion rate) lead gen pages.
In addition, if you’re an early stage startup and you’re still doing customer development, it’s too early for MVT. You may end up with the best performing page, but you won’t learn much. By doing everything at once, you miss out on the ups and downs of understanding the behavior of your audience.
That said, there are definitely some high-impact use cases for MVT.
When should you use a multivariate test?
Multivariate tests are about measuring interaction effects between independent elements to see which combination works best. As Ton Wesseling, founder of Online Dialogue, put it:
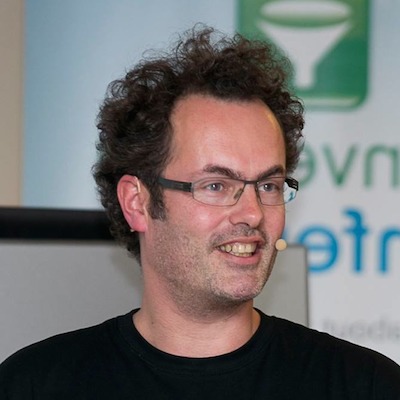
Ton Wesseling:
“When to use MVT? There’s only one answer: if you want to learn about interaction effects. An A/B test with more than one change could not be winning because of interaction effects. A winning new headline could be unnoticed because the new hero shot is pointing attention to a different location on the page. If you want to learn real fast which elements on your page create impact: do a MVT with leaving in and out current elements.”
Paras Chopra from VWO said he’d use MVT for optimizing several variables, but not expecting a huge lift. More for incremental improvements on multiple elements:
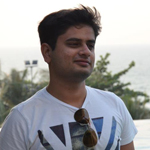
Paras Chopra:
“I’d use multivariate test when I’m doing optimization with several variables, not hoping for a wild swing (that we expect in A/B test). I think the right way is to use A/B test for large changes (such as overhauling entire design) and such. A/B test could be followed up with MVT to further optimize headlines, button texts, etc.”
The benefits of multivariate tests
MVT is awesome for follow-up optimization on the winner from an A/B test once you’ve narrowed the field.
While A/B testing doesn’t tell you anything about the interaction between variables on a single page, MVT does. This can help your redesign efforts by showing you where different page elements will have the most impact.
This is especially useful when designing landing page campaigns, for example, as the data about the impact of a certain element’s design can be applied to future campaigns, even if the context of the element has changed.
Andrew Anderson, Head of Optimization at Malwarebytes, explained that MVT is used to figure out what the most influential item on the page is and then going much deeper on it:
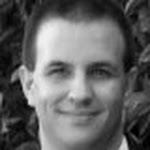
Andrew Anderson:
“It is not about ‘I want to see what happens with three pieces of copy, four images, and a small CTA.’ The question should be what matters most, the copy, the image, or the CTA, and whatever matters most I am going to test out 10 versions (and learn something important).”
A/B testing can never tell you influence, MVT can when it is done right. ANOVA analysis gives you mathematical influence, or the relative amount one factor influences behavior relative to others.”
So a big goal of multivariate testing is to let you know which elements on your site play the biggest role in achieving your objectives.
ANOVA? A quick definition
ANOVA (analysis of variance) is a “collection of statistical models used to analyze the differences among group means and their associated procedures.”
In simple terms, when comparing two samples, we can use the t-test—but ANOVA is used to compare the means of more than two samples.
If you’re looking to dive deep into ANOVA, here’s a great video tutorial to learn:
So if there are certain use cases for multivariate tests, then there are certain ways to execute them. What are the conditions and requirements of running successful multivariate tests?
Multivariate testing: How to do it right
The one big condition of running MVT: “Lots and lots of traffic,” according to Paras Chopra. Therefore, much of the accuracy in running MVT means understanding traffic needs and avoiding false positives.
Common mistakes with running MVT
Though many of the common mistakes of MVT aren’t unique (many apply to A/B testing as well), some are specific to multivariate methods. But they’re pretty much as you’d guess:
- Not enough traffic;
- Not accounting for increased chance of false positives;
- Not using MVT as a learning tool;
- Not using MVT as a part of a systemized approach to optimization.
1. Not enough traffic
We already talked about traffic above, but to reiterate: MVT requires lots of traffic. Fractional factorial methods mitigate this, but there are some questions as to the accuracy of this method.
The increased traffic requirement also presents the question of how long you should expect this test to go. This is especially true if you’re using MVT as a way to throw things at the wall and see what sticks (inefficient).
One thing you should definitely do is estimate the traffic needed for significant results. Use a calculator like this one.
Leonid from Optimizely discussed ways to get around the need for crazy amounts of traffic, including the fractional factorial method (we’ll discuss more below):
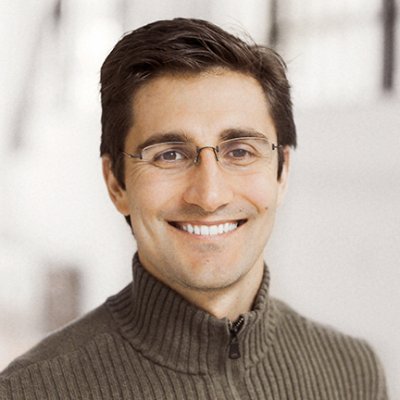
Leonid Pekelis:
“There’s another approach to reducing the need for more visitors in a multivariate test: examine fewer interactions (e.g., only two-way interactions). This is where things like fractional factorial designs come in. You can reduce the required number of visitors by quite a lot if you use fractional factorial instead of full factorial, but you only get to see part of the interaction picture. Things get complicated pretty quickly when you look at all the different design methods out there.
One other use of multivariate tests if you don’t have tons of traffic: start by running a full factorial just to check that none of your changes interact to break your site, you’ll notice those pretty quickly, and then switch to running A/B/n tests to see which changes outperform their baseline.”
Though Matt Gershoff, CEO of Conductrics, said that it’s not necessarily true that an MVT requires more data than would a related set of simple A/B tests. In fact, he says, for the same number of treatments to be evaluated and the same independence assumptions that are implicitly made when running separate A/B tests, an MVT actually requires less data. He continues:
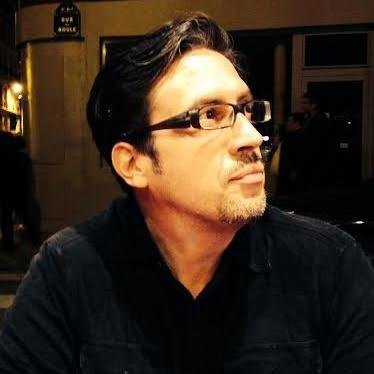
Matt Gershoff:
Regardless of the type of test you decide to run, there are always two steps: 1) data collection; 2) data analysis. One can always collect the data in a multivariate way (full factorial), and then analyze the data assuming that there are no interactions (main effects), or with interactions (we can even pick the degree of the interaction, based on the number of dimensions of the test).
This is why, collecting the data using a full factorial design is nice, because we can analyze it with any degree of interaction we choose—including zero interactions. The only cost, at least in the digital environment, is that we need to have more cells in our database to hold all of the test combinations. If we collect the data in fractional manner our analysis will be constrained based on the nature of the fractional design we used.
Unfortunately, there is no free lunch. Many who balk at the use of main effects MV tests because of concerns about test interactions happily recommend running separate A/B tests—which also implicitly assumes no interaction effects (independence) and requires even more data to evaluate.
2. Not accounting for increased chance of false positives
According to Leonid, the most common mistake in running multivariate tests is not accounting for the increased chance of false positives. His thoughts:
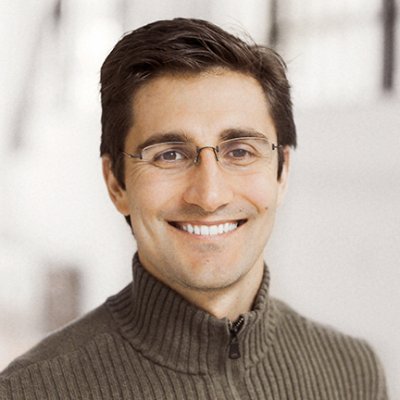
Leonid Pekelis:
“You’re essentially running a separate A/B test for each interaction. If you’ve got 20 interactions to measure, and your testing procedure has a 5% rate of finding false positives for each one, you all of a sudden expect about one interaction to be detected significant completely by chance.
There are ways to account for this, they’re generally called multiple testing corrections, but again, the cost is you tend to need more visitors to see conclusive results.”
We’ve written about multiple comparison problems before. Read a full account here.
3. Not using MVT as a learning tool
As we mentioned in a previous article, optimization is really about “gathering information to inform decisions.” MVT is best used as a learning tool. Using it as a way to drive incremental change and throw stuff at the wall is inefficient and takes time away from more impactful A/B tests. Andrew Anderson put it well in an article on his blog:
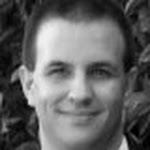
Andrew Anderson:
“The less you spend to reach a conclusion, the greater the ROI. The faster you move, the faster you can get to the next value as well, also increasing the outcome of your program. What is more important is to focus on the use of multivariate as a learning tool only, one that was used to tell us where to apply resources. One that frees us up to test out as many resources for feasible alternatives on the most valuable or influential factor, while eliminating the equivalent waste on factors that do not have the same impact. The goal is to get the outcome, getting overly caught up in doing it in one massive step as opposed to smaller easier steps, is fool’s gold.”
4. Not using MVT as a part of a systemized approach to optimization
Similarly, many MVT mistakes come from people not knowing what they’re planning on doing, or having a testing plan at all. As Paras Chopra put it:
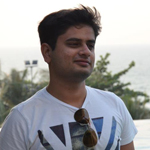
Paras Chopra:
“The biggest mistake is not knowing what they expect out of an MVT. Are they expecting to see best combination of changes or they want to know which element (headline, button) had the maximum impact?”
Andrew Anderson puts it in perspective, saying if you’re using either A/B or MVT testing just to throw stuff against the wall or to validate hypotheses, this will only lead to a personal optimum (i.e. ego-fulfillment). He continues, saying that “tools used correctly to maximize results and maximize resource allocation for future efforts lead to organizational and global maximum.”
Now, I mentioned above that there were different statistical methods for MVT. There’s a bit of a debate between them. Does it matter?
The 3 methods of multivariate testing
There are a few different methods of multivariate testing:
- Full factorial;
- Fractional factorial;
- Taguchi.
There’s a bit of an ideological debate between the methods, as well.
Full factorial multivariate testing
A full factorial experiment is “an experiment whose design consists of two or more factors, each with discrete possible values or “levels,” and whose experimental units take on all possible combinations of these levels across all such factors.”
In other words, full factorial MVT tests all combinations with equal amounts of traffic. That means that it:
- Is more thorough, statistically;
- Requires a ton of traffic.
Paras Chopra wrote in Smashing Magazine a while ago:
“If there are 16 combinations, each one will receive one-sixteenth of all the website traffic. Because each combination gets the same amount of traffic, this method provides all of the data needed to determine which particular combination and section performed best. You might discover that a certain image had no effect on the conversion rate, while the headline was most influential. Because the full factorial method makes no assumptions with regard to statistics or the mathematics of testing, I recommend it for multivariate testing.”
Fractional factorial multivariate testing
Fractional factorial designs are “experimental designs consisting of a carefully chosen subset (fraction) of the experimental runs of a full factorial design.”
So fractional factorial experiments test a sample set by showing significant combinations. Because of that, they require less traffic:
Though, an Adobe blog post likened fractional factorial design to a barometer, saying “a barometer measures atmospheric pressure, but its value is not so much in the precise measurement as the notification that there is a directional change in pressure.”
The same article then also said:
I question how valuable it is to spend five months running one single test for learnings that may no longer be applicable by the time the test has completed and the data pumped through analysis. Instead, why not take the winnings and learnings of your week-long fractional-factorial multivariate test and then run another test that builds off that new and improved baseline?
Taguchi multivariate testing
This is a bit more esoteric, so it’s best not to worry about it. As Paras wrote in Smashing Magazine:
It’s a set of heuristics, not a theoretically sound method. It was originally used in the manufacturing industry, where specific assumptions were made in order to decrease the number of combinations needing to be tested for QA and other experiments. These assumptions are not applicable to online testing, so you shouldn’t need to do any Taguchi testing. Stick to the other methods.
Further reading: Taguchi Sucks for Landing Page Testing by Tim Ash
So does it matter?
As mentioned above, most of the debate lies in the murkier statistics of the fractional factorial method. A large amount of the optimizers I talked to said they only recommend full factorial. As Paras explains, “A lot of ‘fractional factorial’ methods out there are pseudo scientific, so unless the MVT method is properly explained and justified, I’d stick to full factorial.”
However, some, like Andrew Anderson, hold that these debates in general are misguided. As he explains:
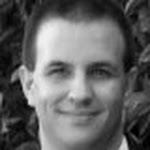
Andrew Anderson:
“Debating which is better, partial or full factorial, at that point is useless because you are just arguing over what shade of green is one leaf in the large forest. MVT should be used to look for influence and focus future resources, in which case it is just a fit and data accessibility question. Any other use of MVT missed that boat completely and just highlights the lack of discipline and understanding of optimization.”
So does it really matter? I don’t know. If you have enough traffic, I think full factorial is harder to mess up. That said, you’re making business decisions that are time critical, so if a full factorial test will take you six months to complete, it’s probably not worth the accuracy.
Conclusion
If you have enough traffic, use both types of tests. Each one has a different and specific impact on your optimization program, and used together, can help you get the most out of your site. Here’s how:
- Use A/B testing to determine best layouts.
- Use MVT to polish the layouts to make sure all the elements interact with each other in the best possible way.
As I said before, you need to get a ton of traffic to the page you’re testing before even considering MVT.
Test major elements like value proposition emphasis, page layout (image vs. copy balance, etc.), copy length and general eyeflow via A/B testing, and it will probably take you 2-4 test rounds to figure this out. Once you’ve determined the overall picture, now you may want to test interaction effects using MVT.
However, makes sure your priorities align with your testing program. Peep once said, “most top agencies that I’ve talked to about this run about 10 A/B tests for every one MVT.”
Great post, many thanks.
To add to the complexity of this discussion: What approach do you recommend for testing something like a loan calculater with different possible default values?
– A/B/N Test in parallel, e.g. one round with A=control vs. B=different default in field 1 vs. C=different default in field 2 vs. B+C
– A/B/N Test in sequence, e.g. 1st round: A=control vs. B, 2nd round: Winner 1st round vs. C
– MVT is probabely not applicable since you can’t hide fields completely
Hi Alex,
Its helpful reading about the pros and cons of the A/B test and Multivariate Tests. The article is detailed and its cool learning new insights from it. At least I now understand what it takes to do a multivariate test. The examples are revealing!
Having said that, I think my best takeaway in this post comes from the concluding part:
I left the above comment in kingged.com as well
Hey nice article. Interesting to see how different people approach it.
I want to mention that ANOVA would be a rather crude tool for analyzing the results of an MVT. It is only a comparison between means, so a lot of information is being lost. Additionally, conversions are a binary variable and with ANOVA you are comparing their transformations (a conversion rate), meaning you lose some signal. These problems show up huge in your DoE when calculating sample sizes – you need some ridiculous numbers there. To top all of this off, comparing means will only show you if there are differences in the distributions, not how big they are. Many people miss this and this leads to a lot of ridiculous blog posts claiming insane conversion lifts, which are simply not there. If you chose to include effect sizes, that will make your DoE even more complex, but worse – you risk losing a lot of information, especially in a multivariate scenario like this one.
Some other ways to overcome the above problems:
1) Using MANOVA (https://en.wikipedia.org/wiki/Multivariate_analysis_of_variance) instead. This is an extension of ANOVA meant specifically for such scenarios. It will fix the sample sizes problem up to a point. Additionally, you can include other variables besides the testing elements – (i.e. channel) to unlock even more insight, however those need to have some logic behind them or you risk overfitting.
2) Logistic regression. That would be my go-to tool for the job. It addresses all the problems I outlined and you can add addition variables in it too (i.e. channel). You can quantify the effect every component/combination has, which is simply not possible with means tests. There are other pros, like for example testing only major variations and getting info about combos you did not think of and allocating more sample to them mid-flight. You can go full-nuts mode and test incredibly diverse scenarios using a nested extension, if your heart desires.
3) CART would be an especially good option for the scenarios Andrew Anderson mentions.
Simple ANOVA would be the tool I chose for A/B/n tests in simultaneous flight. A lot of people mention how you should only be having 1 test on a page, because of poor performance of t-tests. That’s the answer they are looking for.
One thing I want to add is that when doing factorial designs you most definitely need to screen the combinations. More often than not there would be some that simply don’t make sense, so you can save a lot of testing sample by removing them.
Would love to know what you think or want to expand on something.
(I wrote this post on GH thread first, but it was considered spam, so I figured I’d post it here instead)
Hi Momchil -long time ;)
I am not sure I fully understand your comment, especially the suggestion to use MANOVA, but let me take a crack at it. I think you are raising three possible issues:
1) Limited Dependent Variables: When running tests, you can have different measures: continuous outcomes (like sales amount per order), count (0,1,2,3…) , and binary (convert (yes, no)) etc. If you have a binary dependent variable, then logistic regression can be a good choice, since 1) it outputs probability scores (in the range of {0,1}), and, and this is really not that important, removes a source of heteroscedastic errors that fitting a simple linear model will suffer from. For online testing, I am not sure of how much extra value this is, since one will now have the issue of explaining/interpreting log odds. That might be a deal breaker for most organizations.
2) Multiple correlated Dependent Variables: I am not sure if this is what you were getting at, but since you mentioned MANOVA, I am assuming that is what you mean. Most folks are not looking to test multiple outcome variables jointly, but yeah, you could do this, but MANOVA, like ANOVA, is going to assume homoscedastic error terms. So it won’t solve the limited dependent variable issue, if that is something you are really worried about.
3) Control for nuisance / contextual independent variables (this is your ‘channel’ example)– if there are external aspects of the problem domain outside of our direct control, that can explain variance in our outcome variables, we can improve our results by accounting for them directly in our model. This is something that one could do with either an ANCOVA model, or with direct regression approaches. As you know, ANOVA, is essentially analogous to linear regression with dummy variable encodings of the treatment effects. ANCOVA is basically the same thing, but allows for the inclusion of continuous variables, along with our treatment variables, and is just like linear regression with the dummy treatment encoding and added continuous variables on the right hand side – Outcome=f(treatment(dummy) + ‘channel’ + ‘day part’ + ‘device type’ ….).
If you wanted to blend this stuff together, I guess you could us MANCOVA, or even use Zellner’s Seemingly Unrelated Regression (SUR) – the error structure across equations is assumed to be correlated, or whatever is in use today (not my expertise). I guess it depends on what you want to test really.
I am afraid I don’t see how CART/CHAID etc is going to be of much use for testing. It for sure can be useful for predicting and learning a mapping between customer attributes and outcomes. In fact back in the ‘90s when I was in Database Marketing, I would often use a tree model rather than logistic regression, just because clients often never really understood the regression model, and without understanding, you almost never got client buy in – which is true today, and I think the main take away.
All that said, for most basic test situations, it is hard for me to see when using a factorial ANOVA isn’t going to be robust enough come up with a good result in almost all basic testing situations (esp since under the cover it is essentially linear regression.) All else being equal, East to West, Least Squares is BEST ;-)
Hey Alex,
Great information here, but the details went over my head LOL
But I didn’t know about MVT. I use A/B testing for my opt in forms on my blog and it looks like I had incorporated MVT. I run a contest between forms and which form gets the most opt ins, I’ll use it, copy the form, and make minor changes. This is where the MVT comes in.
But according to your article, on average a site should be getting 100,000 unique visitors/month in order to use MVT efficiently. I’m far from having stat this per month and I might be confused about the difference between A/B testing versus MVT.
Thanks Sherman, glad you liked the article. If you’re not getting enough traffic, I wouldn’t worry about MVT – a solid a/b testing program should suffice.
Here’s a good article if you want to read more on the topic: https://cxl.com/how-to-build-a-strong-ab-testing-plan-that-gets-results/