Sometimes A/B testing is made to seem like some magical tool that will fix all problems at once. Conversions low? Well run a test and increase your conversions by 12433%! It’s easy!
Setting up and running split tests is indeed easy (if you’re using the right tools), but doing it right requires thought and care.
Table of contents
1. Most A/B tests won’t produce huge gains (and that’s okay)
I’ve read the same A/B testing case studies as you have. Probably more. One huge gain after another – or so it seems. The truth is that vast majority of tests are never published. Like most people who try to make it in Hollywood are people you won’t ever hear about, you don’t know about most A/B tests.
Most split test “fail” in the sense that they won’t result in a lift in conversions (new variations produce either no change or perform poorer). Appsumo founder Noah Kagan has said this about their experience:
Only 1 out of 8 A/B tests have driven significant change.
It’s a good expectation to have. If you’re expecting every test to be a home run, you’re setting yourself up for some unhappy times.
Think of it as process of continuous improvement
Conversion optimization is a process. And improving conversions is like getting better at anything – you have to do it again and again. It often takes many tests to gain valuable insights about what works and what doesn’t. Every product and audience is different, and even the best research will only take us so far. Ultimately we need to test our hypothesis in the real world and gain new insights from the test results.
It’s about incremental gains
A realistic expectation to have is that you’ll achieve a 10% gain here and 7% gain there. In the end all of these improvements will add up.
“Failed” experiments are for learning
I’ve had a ton of cases where I came up with a killer hypothesis, re-wrote the copy and made the page much more awesome – only to see it perform WORSE than the control. Probably you’ve experienced the same.
Unless you missed some critical insights in the process, you can usually turn “failed” experiments into wins.
I have not failed 10,000 times. I have successfully found 10,000 ways that will not work.
– Thomas Alva Edison, inventor
The real goal of A/B tests is not a lift in conversions (that’s a nice side effect), but learning something about your target audience. You can take those insights about your users and use it across your marketing efferts – PPC ads, email subject lines, sales copy and so on.
Whenever you test variations against the control, you need to have a hypothesis as to what might work. Now when you observe variations win or lose, you will be able to identify which elements really make a difference.
When a test fails, you need to
- evaluate the hypotheses,
- look at the heat map / click map data to assess user behavior on the site,
- pay attention to any engagement data – even if users didn’t take your most wanted action, did they do anything else (higher clickthroughs, more time on site etc).
2. There’s a lot of waiting (until statistical confidence)
A friend of mine was split testing his new landing page. He kept emailing me his results and findings. I was happy he performed so many tests, but he started to have “results” way too often. At one point I asked him “How long do you run a test for?” His answer: “until one of the variations seems to be winning”.
Wrong answer. If you end the test too soon, there’s a high chance you’ll actually get wrong results. You can’t jump to conclusions before you reach statistical confidence.
Statistical significance is everything
Statistical confidence is the probability that a test result is accurate. Noah from 37Signals said it well:
Running an A/B test without thinking about statistical confidence is worse than not running a test at all—it gives you false confidence that you know what works for your site, when the truth is that you don’t know any better than if you hadn’t run the test.
Most researchers use the 95% confidence level before making any conclusions. At 95% confidence level the likelihood of the result being random is very small. Basically we’re saying “this change is not a fluke or caused by chance, it probably happened due to the changes we made”.
If the results are not statistically significant, the results might be caused by random factors and there’s no relationship between the changes you made and the test results (this called the null hypothesis).
Calculating statistical confidence is too complex for most, so I recommend you use a tool for this.
Beware of small sample sizes
I started a test for a client. 2 days in, these were the results:
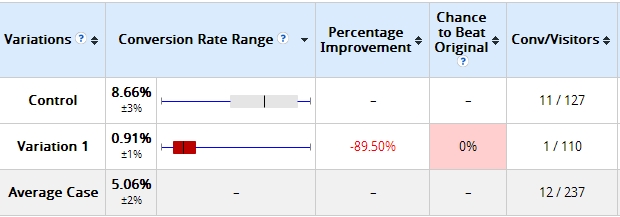
The variation I built was losing bad – by more than 89%. Some tools would already call it and say statistical significance was 100%. The software I used said Variation 1 has 0% chance to beat Control. My client was ready to call it quits.
However since the sample size here was too small (only a little over 100 visits per variation) I persisted and this is what it looked like 10 days later:
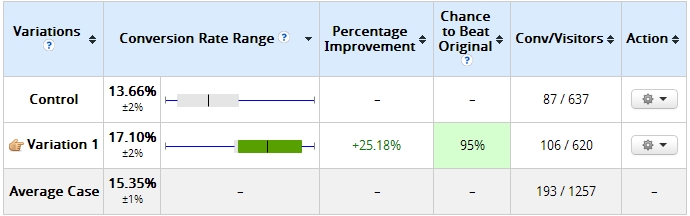
That’s right – the variation that had 0% chance of beating control was now winning with 95% confidence.
Don’t make conclusions based on a very small sample size. A good ballpark is to aim for at least 100 conversions per variation before looking at statistical confidence (although a smaller sampler might be just fine in some cases). Naturally there’s a proper statistical way to go about determining the needed samples size, but unless you’re a data geek, use this tool (it will say statistical confidence N/A if proper sample size not achieved).
Watch out for A/B testing tools “calling it early”, always double check the numbers. Recently Joanna from Copy Hackers posted about her experience with a tool declaring a winner too soon. Always pay attention to the margin of error and sample size.
Patience, my young friend
Don’t be discouraged by the sample sizes required – unless you have a very high traffic website, it’s always going to take longer than you’d like. Rather be testing something slowly than to testing nothing at all. Every day without an active test is a day wasted.
3. Trickery doesn’t provide serious lifts, understanding the user does
I liked this tweet by Naomi Niles:
Next time I see an article telling people to increase their conversion rate by using one color instead of another, I’m going to cry.
— Naomi Niles (@NaomiNiles) December 10, 2012
I couldn’t agree more. This kind of narrative gives people the wrong idea about what testing is about. Yes sure – sometimes the color affects results – especially when it affects visual hierarchy, makes the call to action stand out better and so on. But “green vs orange” is not the essence of A/B testing. It’s about understanding the target audience. Doing research and analysis can be tedious and it’s definitely hard work, but it’s something you need to do.
In order to give your conversions a serious lift you need to do conversion research. You need to do the heavy lifting.
Serious gains in conversions don’t come from psychological trickery, but from analyzing what your customers really need, the language that resonates with them and how they want to buy it. It’s about relevancy and perceived value of the total offer.
Conclusion
1. Have realistic expectations about tests.
2. Patience, young grasshopper.
3. A/B testing is about learning. True lifts in conversions come from understanding the user and serving relevant and valuable offers.
[grwebform url=”https://app.getresponse.com/view_webform_v2.js?u=Td&webforms_id=6203803″ css=”on” center=”off” center_margin=”200″/]
Hi,
Do you know what is the test performed behind mystatscalc (geek stuff)?
Im not sure about this site in particular, but theres lots of statistical confidence algorithm code samples around if google a bit
Hi, good post. What tools are you using. I’m thinking of the two screenshots of statistical data. Doesn’t seem like it is mystatscalc.com.
— christian
This is Visual Website Optimizer, I use this for split testing
Nice read. Point 2 is very important, a lot of results of A/B tests I ran changed after the first days. Never make conclusions to fast, don’t follow your gut, only follow statistical proof.
Exactly!
As an addition to Jente’s point it might be worth running tests with less variables so that you can reach the sample size that you want. Taking time to really plan and develop the content being tested can save time in the long run! :)
As you say, Analysis and understand what are you doing, and what your visitors are doing in your site is the key to improve your CR.
I keep an eye on the tool, Visual Website Optimizer
Thanks!
Every client and new CRO-tester in the world should read this post. You did a great job at putting things simply. I am going to use this article as a reference to explain CRO to prospective clients.
Thanks Bryant
I’m glad you pointed these out, Peep! And I’m also glad to hear I’m not the only one who has these types of frustrations with commonly held beliefs.
Great post – I don’t think http://mystatscalc.com/ is reliable to calculate the confidence to beat the control, if you use it with the data from your screenshot, you will find out a 90.89% chance to beat control, where your tool show 95% (with my formula I found 95.46%).
Anyway I think your conclusion is very true – it is all about the learning…and patience!
Great Post, The ab test is essential today for a marketing strategy long term oline serious, always thinking about the conversion.
Good post Peep. Sadly, things like patience, realistic expectations and a thorough process of audience understanding (which do work) are very much out of fashion in today’s one-click solution (that doesn’t work) environment. Keep up the good work.
Thanks for sharing. We should take care with the % of the improvement if that is calculated on other percentages. I mean, % of improvement of 0.5% to 1% is 100%, looks like a completely success, when really is a logical trend when you are improving from the worse scenario.
The AB test is essential today for a marketing strategy long term oline serious. Great Post ;)
Thanks for sharing Peep! We use Google’s A/B testing tool and you are correct with your conclusion, A/B testing takes time and patience and lot’s of small tweaks and testing ;-)
It’s an amazing piece of writing designed for all the online people; they
will obtain benefit from it I am sure.
Hi, I think you’ve reach the point by saying that at the end A/B test is about understanding your audience more than trying this colour or that picture size instead of the other. Only by really focusing on users, trying to gain knowledge of them by our test, one can finally increase conversion, but sadly most of the company thing backwards, targeting a goal and then by making small changes hope that they get there.
Thanks for sharing Peep! We use Google’s A/B testing tool and you are correct with your conclusion, A/B testing takes time and patience and lot’s of small tweaks and testing ;-)
So true. A/B Testing it’s a necessary item in the checklist but the improvement not finish there! thank for sharing the post with this humor. ;)
Thanks for sharing Peep! We use Google’s A/B testing tool and you are correct with your conclusion, A/B testing takes time and patience and lot’s of small tweaks and testing ;-)
Great Post, The ab test is essential today for a marketing strategy long term oline serious, always thinking about the conversion.
A nice article and some nice tips. I think, however, that statements such as “statistical significance is everyting” or recommending a 95% significance as a rule of thumb is not very helpful.
The first can make a person forget about statistical power and fall prey to arbitrary stopping (e.g. testing ends when reached signficance) which can basically increase your error rate from 5% to 100%. I argue strongly and in detail about those issues here: http://blog.analytics-toolkit.com/2014/why-every-internet-marketer-should-be-a-statistician/
The second thing is that 95% significance might be too high a price to pay in case of small risk/cost to test. Also, it might be far too uncertain if the stakes are high or the decision – irreversible!
Useful post Peep!, we tried A/B testing on all our major campaigns and final what we felt is.. Google revenue increased and we have wasted lot of money in testing the adcopies.
A/B testing is for sure help us in improving our website to be more attractive to our readers.
Nice article! really tookme like a half hour to undestand but… now im like NEO on Matrix ehehe